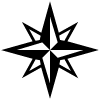
@ LessWrong (RSS Feed)
2025-04-28 06:42:48
Therapist in the Weights: Risks of Hyper-Introspection in Future AI Systems
Published on April 28, 2025 6:42 AM GMTEpistemic Status: Speculative, but grounded in current trends in interpretability and self-supervised learning. This was initially written as an outline at some point in 2024, but written/rewritten more fully in response to Anthropic’s announcement that it was pursuing this general direction. (Drafting was assisted by GPT4o, but the direction and rewriting to fix its misunderstandings are my own.)Background and Motivation - What is Hyper-Introspection?Most alignment conversations treat LLMs as black boxes that can be prompted, steered, or fine-tuned by external agents. But as alignment research increasingly focuses on interpretability, and is increasingly co-managed by the systems themselves, it seems that the safety plan isn’t just better outer control—but better inner transparency. That is, we imagine LLMs that can not only tell you when they’re uncertain or wrong, but accurately identify why are where they went wrong. That might include what prior associations led to the mistake, where in the weights the issue is, or what training data shaped the answers in a given direction.This post posits and explores this idea of hyper-introspection, which is that future models might develop or be given the capacity to explicitly inspect themselves, create some implicit clear self-model that is based on this information, detect and debug their own reasoning chains, and rather than the oft-noted concerns about designing their successor, will propose or implement self-modifications. This would be intended to allow error detection, causal traceability, and self-correction all bundled into a system that can self correct, notice when it is failing, and propose changes.But this isn’t just promising—it’s also incredibly risky. Giving models the tools to model and modify themselves could accelerate both prosaic near-term alignment, the focus on Anthropic's current interpretability research, and also deceptive longer-term misalignment. Our understanding of the systems is crude enough that we are unlikely to detect when systems are misaligned, or becoming misaligned, especially when they have a better understanding of themselves than the humans overseeing them. We don't have anything better than rough heuristics for when and why fine-tuning works, and we should expect that we won't have any better understanding when the model is being used for the analysis or for proposing changes.Why is this Different?Currently, LLMs can sometimes notice contradictions or errors via chain-of-thought prompting, or when asked to double check their work. But successful self-correction without pretty explicit prompting on the part of humans is not common, and is certainly not reliable. The models lack self-awareness to realize how to introspect, much less the ability to meaningfully access their own training history or weights, so they are not only a black box, but one they cannot even see. In some cases, researchers with access to the weights have tools that allow them to interpret the model’s actions, but the model itself does not use those tools. Similarly, researchers can access things like relative probabilities of tokens, but the model itself only “sees” the tokens which are realized.Current research on interpretability is essentially capabilities-focused, including work on detecting inconsistencies and hallucinations to improve the model’s performance. Interpretability tools can be used to that end, though I’m unsure how or whether this is done in practice for reasoning models, and in general the approach seems to be to use such tools only during training. However, even without interpretability tools, it seems that models have some degree of functional introspective ability. (For example, early testing showed that models seem to have a rudimentary and low-accuracy but predictive ability to estimate the temperature of a generated output.)I’m positing future work that enables what I will call Hyper-Introspection, a capability set encompassing at least tool usage to inspect its own weights and perform interpretability work on them, which is then used to diagnose and understand its behavior. This might be used for the detection of internal contradictions or failures, and tracing such issues or failures back to latent associations, subnetworks, or training examples. Essentially, this would look something like a combined debugger, therapist, and historian living inside the model.Analogy / Intuition Pump:I spent at least a decade absolutely certain that I hated mushrooms. At some point, I connected this in my mind to the story that I had heard countless times about how, when I was three, I ate a mushroom in the back yard, my sister ran and told my parents, and I had my stomach pumped. At some point, I realized my dislike of mushrooms presumably dated to that event, and actually tried some. In fact, I now really like mushrooms.More generally, a hyper-introspective human might notice some preference or trait that they don’t like, trace it back to childhood experiences, or books they read, or some other factor, and then actively reshape their mental model. Now imagine that—but as an internal loop running inside an LLM-based system.Defining Hyper-Introspection More PreciselyWhat I mean when I talk about "hyper-introspection" is models that can access, analyze, and explain their own internal workings with enough fidelity that they can trace behaviors to causes, and recommend changes, or more concerningly, intervene on their own weights.More precisely, hyper-introspection would include at least low level access, the capability to diagnose or research specific cases, and the ability to diagnose and propose changes.Low level access means that the model has and uses tools which can query its own internal state — not just outputs or token-level activations, but weights, attention patterns, activations, gradients. Diagnosing and research cases means that given a particular output, bias, or failure, the model can trace the origin using the low level tools. It would answer questions like "Which subnetworks or weight clusters most contributed to this mistaken conclusion?" or "Was this behavior more likely shaped by pre-training data, fine-tuning, or emergent optimization?" Diagnosis and proposing changes means that the model can propose causal stories about how these internals led to specific behavior - not in the current chain-of-thought post-hoc rationalization sense, but via mechanisms. And based on introspection, the model would suggest (or even implement) targeted changes. For example, removing harmful or spurious associations, adjusting internal weighting between conflicting concepts, or reweighting experts in the mixture of experts. To do this the model would address some amount of the embedded agency problem, most worryingly, to be used in reinforcement learning without clear segregation of goals. That is, it would create and update a working self-model of its own tendencies, failure modes, and likely causes for both, but update that self-model partly to pursue its goals.In other words, hyper-introspection is what you would get if you embedded an interpretability researcher, a debugger, and a slightly neurotic therapist inside the model, with real-time access to the model's own mind, but didn’t give it a way to protect its goals or define that goal clearly. This is a much more dangerous tool than simple confidence estimates or chain-of-thought prompting. It turns a passive black box into something more like an active epistemic agent — one that not only acts, but studies and edits itself.Of course, true hyper-introspection may require architectural changes, not just better prompting or training. But this is exactly the direction that Anthropic seems to be pursuing. Of course, it might not be possible to do, and requires substantial progress on interpretability, but that is what is being planned - alongside current work building the type of agentic system that could implement the types of work I’ve suggested.Given that, I don’t want to suggest more about how this could happen, or why it might be valuable for capabilities. Instead, I will note that there is a case which will likely be made that this capability would be accessible externally, so that hyper-introspection can radically improve oversight. This is deeply dangerous, and leads to almost exactly the same failure mode as OpenAI discusses when https://openai.com/index/chain-of-thought-monitoring/
https://www.lesswrong.com/posts/HHpiDAb3sHerkG93K/therapist-in-the-weights-risks-of-hyper-introspection-in